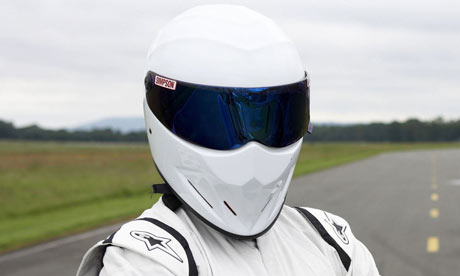
Last week, AWS launched their flavor of "Machine Learning as a service", aka
Amazon Machine Learning. It was not a moment too soon, given the number of existing cloud-based ML propositions. To name just a few: BigML, Qubole and yes, Azure Machine Learning (pretty impressive, I'm sorry to admit).
So, here it is finally. Let's take it for a ride.
First things first: some data is needed. Time to use a little Java program that I wrote to pump out test data simulating an e-commerce web log (see
Generator.java in
https://github.com/juliensimon/DataStuff).
Here's the format, columns are pretty self-explanatory:
Nothing fancy, but it should do the trick.
Next step: connect to my super fancy 1-node Redshift cluster and create an appropriate table for this data:
Next, let's generate 10,000,000 lines, write them in a CSV file and upload it to my favorite S3 bucket located in
eu-west-1. And now the AWS fun begins! Right now, Amazon ML is only available in
us-east-1, which means that your Redshift cluster must be in the same region, as well as the S3 bucket used to output files (as I later found out). Bottom line: if everything is in
us-east-1 for now, your life will be easier ;)
Lucky me, the only cross-region operation allowed in this scenario is copying data from S3 to Redshift, here's how:
For the record, this took just under a minute for 450MB. That's about 100MB per second sustained. Not bad :)
Let's take a quick look:
SELECT * FROM mydata LIMIT 10;
Looks good. Time to fire up Amazon ML. The process is quite simple:
- Create a datasource, either from an S3 file of from Redshift
- Pick the column you want to predict the value for (in our case, we'll use 'basket')
- Send some data to build and evaluate the model (we'll use the 10M-line file)
- If the model is good enough, use it to predict values for new data
Creating the datasource from Redshift is straightforward: cluster id, credentials, table name, SQL statement to build the test data.
Once connected to Redshift, Amazon ML figures out the schema and data types:
Now, let's select our target column (the one we want to predict):
Next, we can customize the model. Since this is a numerical value, Amazon ML will use a
numerical regression algorithm. If we had picked a boolean value, a
different algorithm would have been used. Keep on eye on this in future
releases, I'm sure AWS will add more algos and allow users to tweak them
much more than today.
As you can see, 70% of data is used to build the model,
30% to evaluate it.
Next, Amazon ML ingests the data: In our case, this means 10 million lines, which
takes a little while. You can see the different tasks: splitting the data, building the model, evaluating it.
A few coffees later, all tasks are completed. The longest one was by far building the ML model. The whole process lasted just under a hour (reminder: 10 columns, 10 millions lines).
So, is this model any good? Amazon ML gives limited information for now, but here it is:
That promising "
Explore the model performance" button displays a distribution curve of residuals for the part of the data set used to evaluate the model. Nothing extraordinary.
As a sidenote, I think it's pretty interesting to see that a model can be build from totally random data. What does this say about the Java random generator? I'm not sure.
Now, we're ready to predict! Amazon ML supports batch prediction and real-time prediction through an API. I'll use batch for now. Using a second data set of 10,000 lines missing the '
basket' column, let's build a second data source (from S3 this time):
Two news tasks are created: ingest the data from S3 and predict. After a 3-4 minutes, prediction is complete:
A nice distribution curve of predicted values is also available.
Actual predicted values are available in a gzip'ed text file in S3:
Pretty cool... but one last question needs to be answered. How much does it cost? Well, I did push the envelope all afternoon and so...
Over a thousand bucks. Ouch! Expensive fun indeed. I guess I'll expense that one :D
One thousand predictions cost $0.1. So, the scenario I described (model building plus 10K predictions) only costs
a few dollars (thanks Jamie
@ideasasylum for pointing it out).
However, if you decide to use live prediction on a high-traffic website or if you want to go crazy on data-mining, costs will rise VERY quickly. Caveat emptor. AWS has an history of adapting prices pretty quickly, let's see what happens.
Final words? Amazon ML delivers prediction at scale. Ease of use and documentation are what you'd expect from AWS. Features are pretty limited and the UI is still pretty rough but good things come to those who wait, I guess. Cost rises quickly, so make sure you set and track ROI targets on your ML scenarios. Easier said than done... and that's another story :)
Till next time, keep crunchin'!
(Update: want to learn about real-time prediction with Amazon ML? Read on!)